A Brief Overview of the Genomic Space
The genomic space is currently teeming with a lot of welcomed activity. But there can also be confusion as to which player is doing what. To help provide clarity to more stakeholders, we synthesized a practical overview of the space.
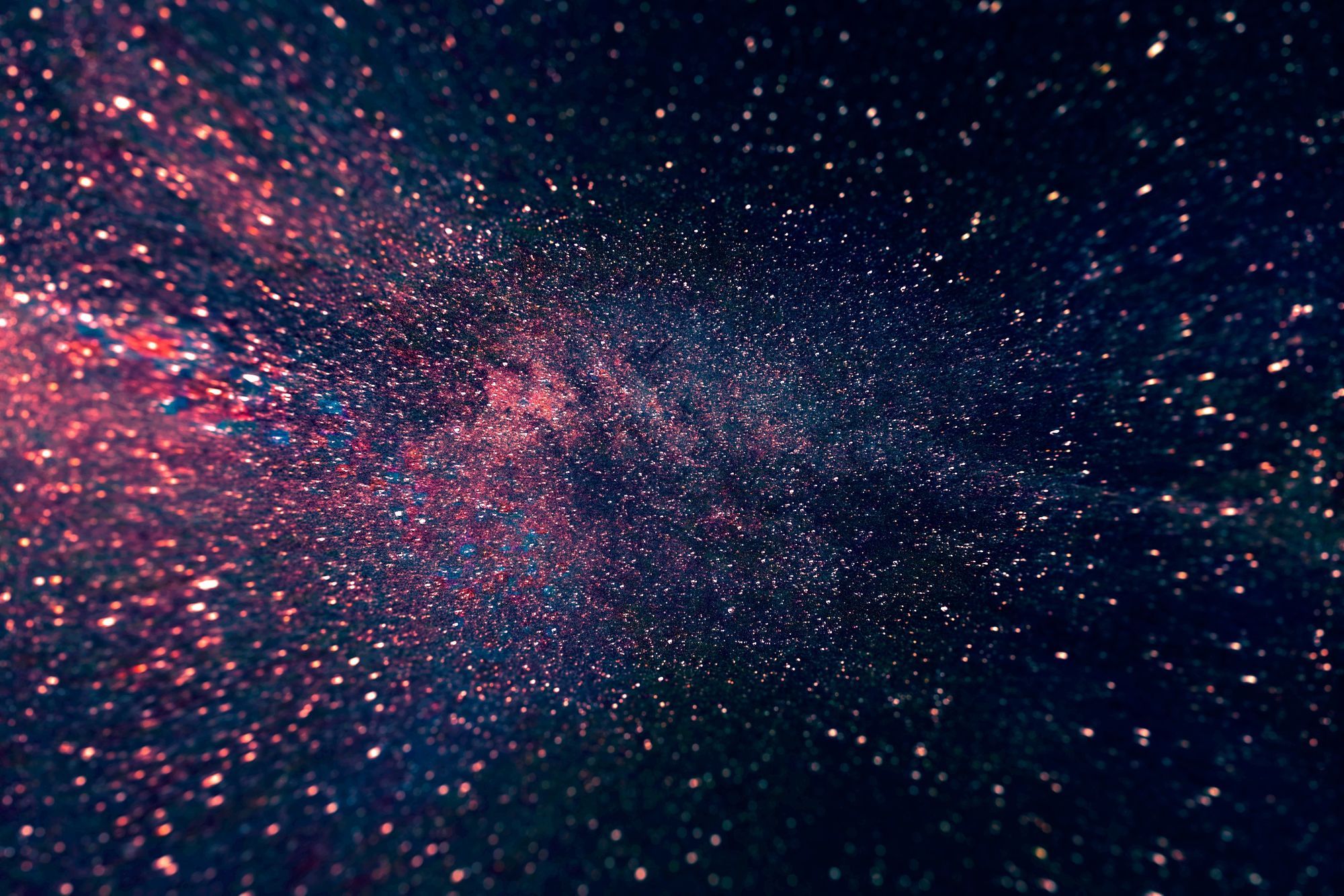
The healthcare space is currently teeming with many ventures endeavoring to improve health outcomes by leveraging the power of the genome, more specifically better genome analysis. This trend is a welcomed development for society. The more people get involved in this noble pursuit, the more likely we are to achieve the needed goals thanks to the collective effort, talent, and resources. Still, care must be taken to ensure that this greater activity remains well-defined and does not decay into chaotic noise, from which could develop confusion that can lead to the slowing or, worse, stoppage of progress. At Genetic Intelligence, we receive many questions from interested parties trying to better understand what we do in relation to other genomic analysis offerings.
With the appreciation that more stakeholders may benefit from additional clarity, we have decided to make our answers to these queries accessible to all:
- In the present post, we'll take a step back to the greater genomic space and break it down into its constituent layers so as to situate everyone well from a high-level perspective.
- Then, in a subsequent post, we will zoom into the layer of the genomic space that is genomic analysis to demystify it and classify the types of genomic analysis performed by the various players.
Overview
The genomic space comprises four main layers: 1) genomic data production, 2) genomic data storage and management, 3) genomic data analysis, and 4) implementation of genomic insights to build products and services.
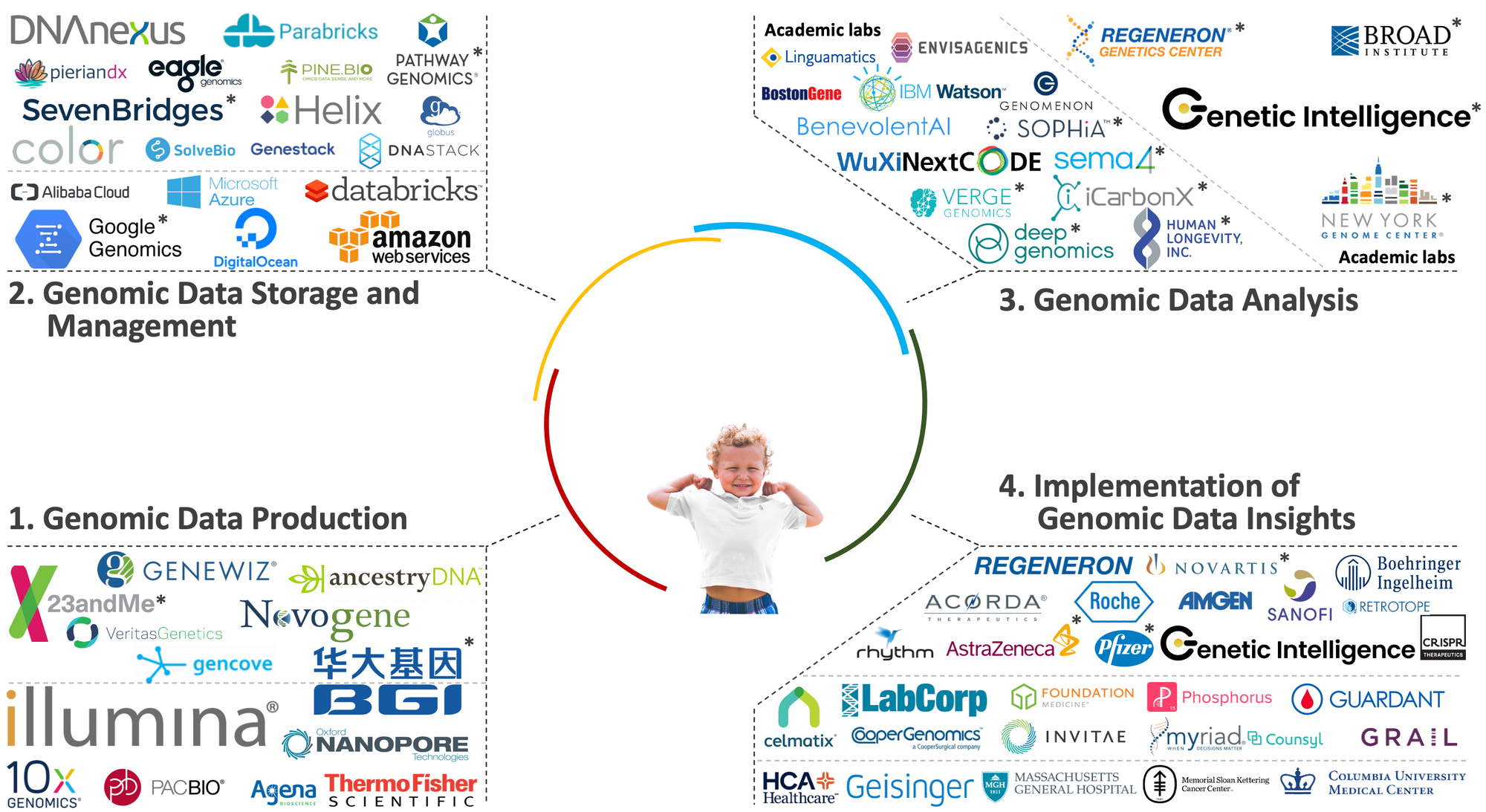
Layer I: Genomic Data Production
It is in this layer that physical DNA is extracted from its biological environs and abstracted into a computational equivalent that can be stored on machines. Recall that the entirety of the DNA found in an organism constitutes its whole genome. This post details the types of genetic data that can be produced from a biological sample since the whole genome is not always sequenced.
There are two main groups in this layer, all working to satisfy the growing demand for sequencing data. The first group is composed of companies that make and sell sequencer machines, a segment that has been categorically dominated by Illumina. The second group is composed of companies that buy sequencer machines to provide genetic sequencing services, for instance 23&Me. A few companies (e.g., BGI) straddle both groups, offering sequencing services both on internally developed machines as well as on machines acquired from outside vendors.
Layer II: Genomic Data Storage and Management
This layer comprises data companies that help others store and process great quantities of genetic data. Several general cloud storage and analytics service companies including Amazon Web Services, Google Cloud, and Databricks are found here, together with more omics-focused cloud companies (e.g., DNA Nexus, SevenBridges) that strive to facilitate and optimize established genomic pipelines while adhering to best practices. Furthermore, some of the companies here also control repositories of genomic data to further empower their platform.
Players in layer II have democratized computational access and continue to lower the requirement for computer science expertise to carry out many genomic analyses. Still, not all genomic ventures make use of offerings in this layer as they may have internal computational clusters, data storage, and/or management systems. Companies that use the typical genome processing and analytics pipeline (i.e., fastq > bam > vcf > gwas > visualization) are more likely to benefit from the optimized version implemented by players in layer II. For instance, even though we often leverage AWS EC2's at Genetic Intelligence, we set up our own pipeline as it is specifically designed to handle the unique features of our platform.
Layer III: Genomic Data Analysis
This layer is where the most progress remains to be achieved, and the greatest value will be unlocked. It has traditionally been the domain of academic research groups and in-house analytics teams at pharmaceutical, biotechnology, and agricultural firms. Increasingly, as the sheer importance of the problem and size of the opportunity here begin to be appreciated by more people together with the increased availability of data and computational access, many new ventures are joining this layer. Some can be extensions of existing, general data analytics companies.
All of these groups in layer III are feverishly working to solve the singular problem of our time: the cypher of the genome function. Genetic Intelligence operates in this layer but internally to the company. The nuances to the strategies and methods employed by various groups in layer III can lead to great confusion. It therefore deserves it's own post where we will look at it in more detail.
An important dichotomy in this layer that is worth mentioning now is that many of the ventures work on better searching, matching, and drawing new insights from the genetic knowledge already sitting in the literature or in variant repositories (i.e., discoveries made and published over the years by scientists). Variations of natural language processing (NLP) artificial intelligence often denote this segment. Meanwhile, a fewer number of ventures focus on innovating better analysis of the genome itself to make new discoveries. It's an important nuance that can be tricky to understand.
Layer IV: Implementation of Genomic Data Insights to build products and services
This layer is incomparably diverse and comprises three main segments: biotechnology/pharmaceutical companies, diagnostics companies, and provider groups (e.g., hospitals and health systems). The burdgeoning predictive health segment also belongs here. Together, they constitute groups that utilize genetic insights produced in layer III to make medical products or render health services. Often, this process involves engaging in drug and diagnostics development, as well as patient care and health management. Health insurance companies would fit into layer IV as well, as they have demonstrated increased interest in genomic data.
It is in this layer that Genetic Intelligence operates, specifically in the biotech/pharma segment by deriving RNA-targeted drugs from its efforts spanning layers II and III.
Further thoughts
This overview of the genomic space is a practical simplification. There are additional aspects that are not included for brevity and to facilitate comprehension. For instance, the following are additional points to consider:
- An important, rather, a critical component of this space that is not shown in this breakdown are the governmental agencies and their genomic data repositories. For instance, the NIH curates dbGaP and is spearheading the All of Us research program that aims to collect data from one million americans, including genomic, lifestyle and health information to fuel the development of precision medicines. There is the UK BioBank and many others. These government-funded repositories liberate genomic data and make it available to all researchers so that a few amassers of genomic data do not impede scientific progress by sitting on data that we believe fundamentally belong to patients. There are also non-profit organizations (e.g., Project MinE) that collect and sequence patient samples to advance research into specific diseases.
- By necessity, many of the companies outside of layer III may perform analytics operations that could be classified as genomic analysis (e.g., layer I to minimize errors or call variants, layer IV for diagnostics), but these are routine analyses and aren't typically the sort of genomic analysis that defines layer III.
- Many companies operate across several segments. For instance, through Verily, Google Genomics, DeepMind, and Deep Brain, Google is attempting to straddle layers II, III, and IV. 23&Me touches all four layers, something HLI and Sema4 also aim to achieve. Similarly, Genetic Intelligence straddles layers II, III, and IV.
- The many companies that provide tools, instruments, or services to collect, preserve and process tissue samples prior to genome sequencing are not mentioned here.
- This overview, especially starting from layer III, is focused on companies addressing human conditions. There are many analogous companies addressing similar problems in animal health, agriculture, livestock and metabolic engineering.