Genetic data samples size: bigger is not better
Most genome analysis efforts are pursuing the “more data, bigger GWAS” strategy to solve the herculean causal gene discovery challenge. But this approach is infeasible on many levels. Rather, actionable disease genes can be identified using a small number of well-structured, well-defined samples.
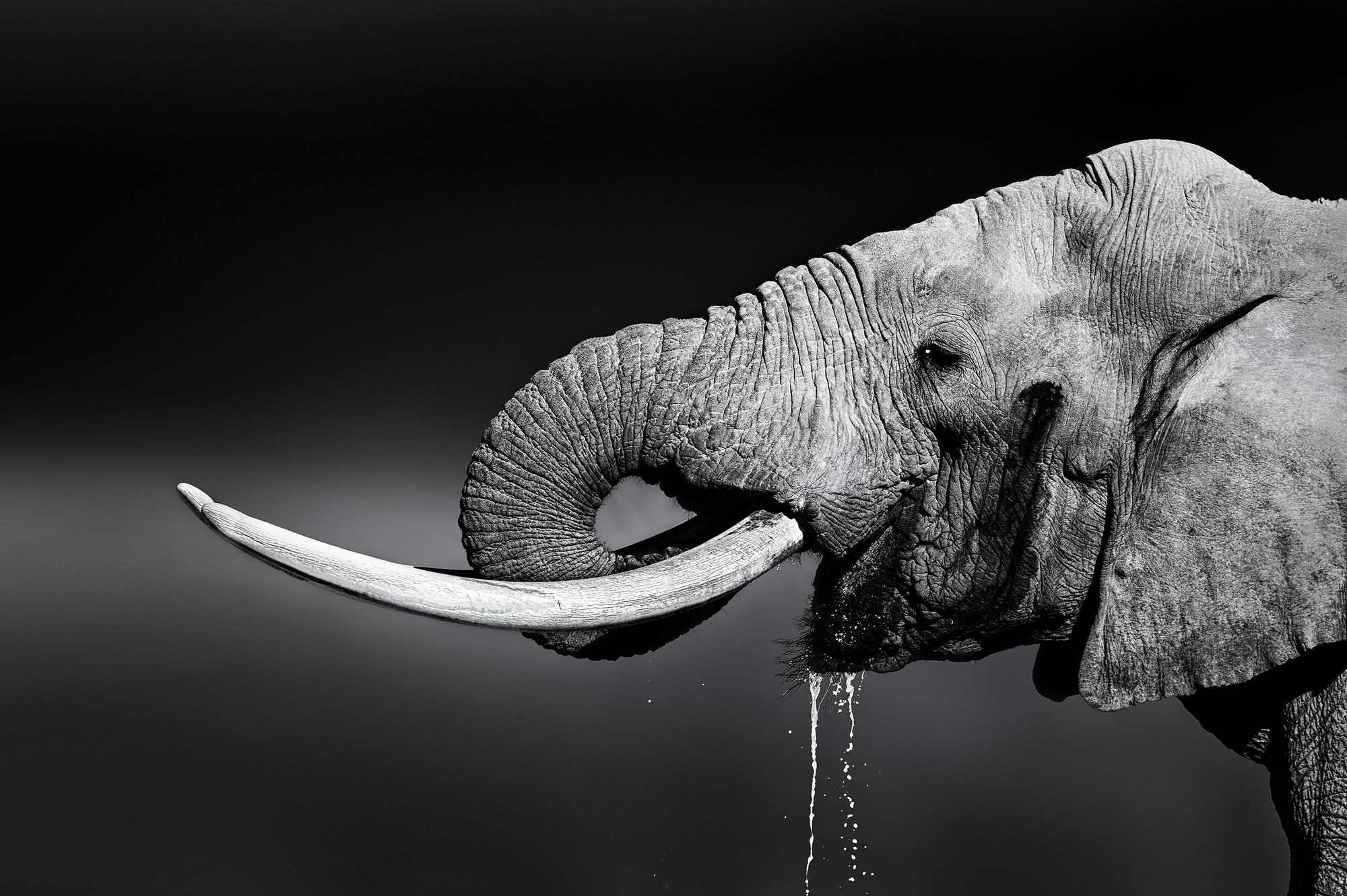
Identifying the causal genetic features of disease is critical for the development of curative medicine and better diagnostics. In a previous post, we discussed why, in the quest to identify the true causal genetic features that underlie disease, it is important to interrogate whole genome sequencing (WGS) data rather than whole exome (WES) data. Here, we tackle the data size (really, the number of samples) question.
The monumental difficulty of finding which specific genetic regions underpin disease cannot be overstated. It is why many years and great resources expended to find these causative genetic factors have yielded few actionable results. In the face of this adverse, persistent reality, the standard answer advanced by researchers is to collect even more samples. To put the moving of goal posts in perspective, the number of target genetic samples to solve the problem has morphed over the years from hundreds to a thousand, then to tens of thousands. The target then became one hundred thousand. It now stands at a few million, and will likely soon become hundreds of million. If the trend holds, at some point we will be told that we must wait until the human population becomes sufficiently large to provide enough statistical power for genetic study.
Here is why genetic researchers and companies push for more samples. Genome wide association studies (GWAS) are the tool of choice that most use to tie disease to causal genes. In a GWAS, genomic data from people with the disease under study is compared to genomic data from people who do not have the disease. Statistical tests are performed to find the probability that the association between a genomic position and the disease is due to chance. Recall that there are 3.5 billion base pair positions in the genomic space in the case of the whole human genome and a few million when only exomic data is concerned. In effect, at least hundreds of thousands of these statistical tests must be performed in a single GWAS and to achieve a high enough statistical power, a great number of samples would be required. Thus, the oft-heard easy answer of the need for more genetic data to finally solve the causal gene discovery problem.
Unfortunately, this straightforward and perfectly understandable answer is incorrect. It will not solve the many, well-documented limitations with GWAS, including difficulty in pointing to true causal mutations, the small effect size of identified associations, and the failure of associations found by one study to be identified by another study even using the same population. All of the limitations of GWAS can be distilled down to the basic statistical precept of type I and type II errors. Intuitively, it is clear that GWAS tends to inflate type I errors (i.e., falsely identifying statistically significant associations that are actually absent). It is why hundreds to thousands of variants are often found to be associated with a trait even though all of this myriad of associations together would only account for a small portion of the trait (i.e., the “missing heritability” problem, which is rather an inflated heritability problem). Endeavoring to lower type I error inflates type II error (i.e., falsely dismissing associations that are actually true). Maintain the higher type I error to keep true associations, and the latter are now lost in a soup of false associations.
Coming back to the point, the many limitations of GWAS are all tied to the fact that the genetic hits identified in these studies are mostly incorrect and non-actionable. More genetic data for even bigger GWAS will not solve this fundamental problem—rather, it will only exacerbate them for two primary reasons.
One, as the genetic data sizes get larger, the statistical significance of many genomic positions will certainly increase. However, the already small effect sizes of these positions will decrease even further as genomic noise is further amplified. The statistical significance identified in a study of small number of samples is more meaningful than the same statistical significance found in a study of large number of samples, especially when the associations found in the latter case are not replicable as is often the case in GWAS studies. This concept is well-explained here. Genomic data is unlike any of the other data types that have benefited from great scale for machine learning (e.g., text and image recognition).
Two, one cannot find millions of people with the specific disease of interest to perform a better structured GWAS. Most diseases don’t have millions of cases (e.g., rare diseases count <200,000 cases) and only a fraction of said patient population will seek genetic testing, much less consent to research. Considering this reality, to achieve higher statistical significance using the heralded hundreds of thousands or millions of samples, samples of differing diseases or conditions must necessarily be mashed up together, exacerbating confounding and the small effect size problem previously discussed.
Others have pointed out these GWAS problems, attempting to sound the alarm that “more data” isn’t the answer. For instance, this paper by researchers at Stanford point out the current problem with the “more data/bigger GWAS” philosophy, offering better study of rare mutations and mapping of regulatory networks as potential solutions. While study of regulatory networks is important, it is only a small part of the solution. What is needed is the ability to identify the few meaningful genetic positions (regulatory or otherwise) that truly denote disease using only a small number of well-structured, well-defined samples. Small in this case means fewer than a few hundred and does not preclude using more data if available and well-characterized. The important point is that causal genetic positions can be identified even when the number of available samples does not number in the hundreds of thousand or millions. This fact not only means better science, it also means lower costs, faster lead times, and better data privacy among many other benefits.
We have now staked two seemingly untenable positions against the current zeitgeist. We maintain that WGS should be analyzed over WES, an intractable challenge in and of itself. We then up the ante by emphasizing that not only should the analysis be of WGS, it should be performed using a small number of well-defined samples, not unattainable and ill-defined millions. In future posts, we will explore how we achieve this goal at Genetic Intelligence.